WETSCAPES2.0
Short profile
Duration
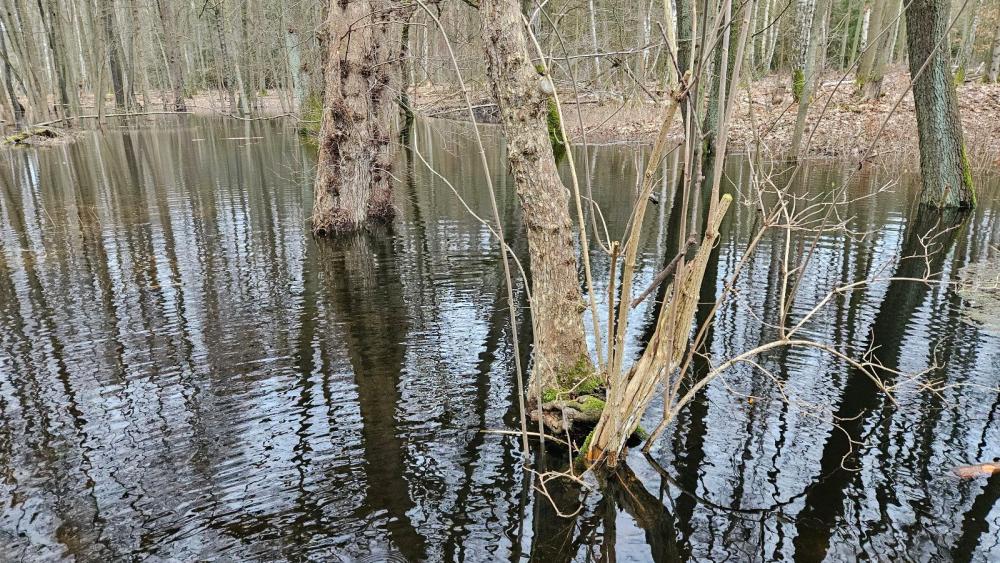
Beautiful wetland | Photo: Dörthe Tetzlaff
Within WETSCAPES 2.0, IGB is leading two projects. The first project “Novel tracer-aided, ecohydrological modeling to quantify water storage, pathways and cycling of fens at the landscape scale” will play an essential integrating role in WETSCAPES2.0 through data-driven, process-based modeling of water cycling, storage dynamics and pathways in restored fens across different spatial and temporal scales. This project will contribute quantifications of spatio-temporal dynamics of water storage, sources, pathways and ages (which provide important hydrological indices of the timescales of water cycling and resilience to perturbation) up to the landscape-scale. The specific objectives are to (i) fundamentally advance and test the capacity of process-based, tracer-aided, ecohydrological modeling frameworks and thus, to (ii) investigate the hydrological functioning of rewetted fens from the plot scale (ca. 10*10m²) up to the landscape scale (ca. 100km2) in terms of spatial patterns and temporal dynamics of water storage, pathways and release.
The 2nd project, “Feedbacks in WETSCAPES2.0: novel, integrated modeling approaches to assess climate change mitigation and adaptation potential” will be conducted in close collaboration with Prof Gerald Jurasinski (University of Greifswald) and Prof Julia Pongratz (LMU Munich). This project will integrate novel data obtained from the monitoring in WETSCAPES2.0 into process-based modeling approaches, with improved model parametrization via data assimilation products and machine learning approaches to quantify physical and biogeochemical process interactions and feedbacks between rewetted peatlands and the landscapes they are imbedded in. The specific objectives are (1) to quantify physical and biogeochemical process-interactions and feedbacks between peatlands, their landscapes and beyond, (2) to identify the challenges and uncertainties in quantifying these feedbacks at larger areas and across scales via multiple, integrated approaches; (3) to use process-based models as learning frameworks by informing and enhancing data collection and experimental setup schemes as well as model structures to reduce uncertainties in the future.
Photo: Dörthe Tetzlaff
Photo: Dörthe Tetzlaff
Photo: Dörthe Tetzlaff
Photo: Dörthe Tetzlaff
University of Greifswald
University of Rostock
Greifswald Mire Centre
GFZ Helmholtz Centre for Geosciences Potsdam
LMU Ludwig-Maximilians-Universität München
Humboldt-Universität zu Berlin
Max Planck Institute for Biogeochemistry
DFG Transregio Collaborative Research Centre 410